Bollywood Movie Recommendations: Math vs. AI (Cosine Similarity!)
Problem Statement:
With the ever-growing number of Bollywood movies, users often struggle to find films that match their preferences. Traditional recommendation methods, such as manual curation or simple genre-based filtering, fail to capture nuanced similarities between movies. This blog explores how mathematical approaches like Cosine Similarity and machine learning models can enhance Bollywood movie recommendations by analyzing factors such as genres, actors, directors, and user ratings. We aim to compare the effectiveness of both approaches in delivering accurate and personalized movie suggestions.
Solving Bollywood Movie Recommendations Using Math (Cosine Similarity)
We will calculate the similarity between two users’ movie preferences step by step using pure math, without programming.
Step 1: Define User Ratings as Vectors
We represent user movie preferences as vectors in n-dimensional space (where each dimension represents a movie).
Let’s assume two viewers Dinesh & Jyoti watched movies (Fighter, Dunki ,Animal , Sam Bahadur and 12th Fail) and gave rating as below table. And based on this we want to recommend movies to another users Manish.
Movie | Fighter (2024) | Dunki (2023) | Animal (2023) | Sam Bahadur (2023) | 12th Fail (2023) |
---|---|---|---|---|---|
Dinesh (A) | 5 | 4 | 5 | 3 | 2 |
Jyoti (B) | 4 | 3 | 4 | 2 | 1 |
Dinesh → A = (5, 4, 5, 3, 2) – Called vector A
Jyoti → B = (4, 3, 4, 2, 1) – Called vector B
Step 2: Apply the Cosine Similarity Formula
The formula for cosine similarity between two vectors A and B is:
cos(θ) = (A ⋅ B) / (‖A‖ ⋅ ‖B‖)where:
- A⋅B = Dot product of A and B
- ∣∣A|| = Magnitude (length) of A
- |B∣∣ = Magnitude (length) of B
Step 3: Compute the Dot Product
The dot product of two vectors is:
Dinesh → A = (5, 4, 5, 3, 2) – vector A
Jyoti → B = (4, 3, 4, 2, 1) – vector B
A⋅B = (5×4)+(4×3)+(5×4)+(3×2)+(2×1) = 20+12+20+6+2=60
Step 4: Compute the Magnitudes
The magnitude of a vector is:
# Magnitude of vector A:
||A|| = sqrt(5² + 4² + 5² + 3² + 2²)
= sqrt(25 + 16 + 25 + 9 + 4)
= sqrt(79) ≈ 8.89
# Magnitude of vector B:
||B|| = sqrt(4² + 3² + 4² + 2² + 1²)
= sqrt(16 + 9 + 16 + 4 + 1)
= sqrt(46) ≈ 6.78
Step 5: Compute Cosine Similarity
# Step 5: Compute Cosine Similarity
cos(θ) = 60 / (8.89 × 6.78)
= 60 / 60.3
≈ 0.995
Step 6: Interpret the Result
Since 0.995 is very close to 1, the two users have very similar movie tastes.
👉 If User 1 liked “Animal”, it is very likely that User 2 will also like “Animal”, so we recommend it to them.
Conclusion: How We Solved This Using Math?
- We represented user ratings as vectors.
- Used cosine similarity formula.
- Computed dot product and magnitudes.
- Found a similarity of 0.995, meaning their movie tastes are very close.
This is the core math behind Netflix & Prime Video recommendations! 🚀
Would you like me to extend this with real IMDb ratings or explore other machine learning metrics? 🎬
Solving Bollywood Movie Recommendations Using Programming Language Python (Cosine Similarity)
from numpy import dot
from numpy.linalg import norm
# Movie ratings for Dinesh and Jyoti
Dinesh = [5, 4, 5, 3, 2] # Fighter, Dunki, Animal, Sam Bahadur, 12th Fail
Jyoti = [4, 3, 4, 2, 1]
# Compute cosine similarity
cosine_similarity = dot(Dinesh, Jyoti) / (norm(Dinesh) * norm(Jyoti))
print("Cosine Similarity:", cosine_similarity)
Output : Cosine Similarity: 0.9953109657524404
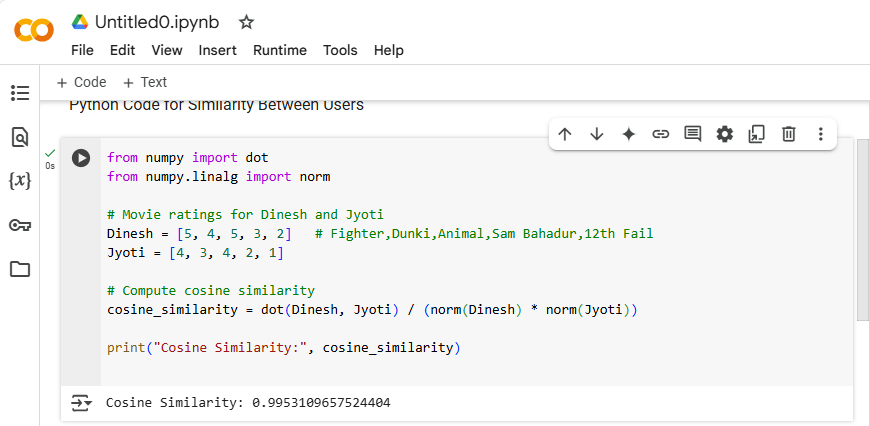
Why It’s Useful?
If the similarity is close to 1, users have similar preferences, and we can recommend movies watched by one user to the other. Now you can see cosine similarity calculation is very easy programmatically. Now we can recommend similar movies to Manish.
Conclusion: Bollywood Movie Recommendations Using Math & Machine Learning 🎬📊
In this example, we used mathematical metrics (cosine similarity) to analyze movie preferences and recommend Bollywood films based on user ratings.
Key Takeaways:
- Mathematics in Recommendations – By treating user movie ratings as vectors and applying cosine similarity, we measured how closely two users’ preferences aligned.
- Machine Learning in Action – In real-world applications, this concept extends to collaborative filtering in machine learning, where algorithms recommend movies based on patterns in user behavior.
- Bollywood Movie Example – If two users rated movies like Animal (2023) and Dunki (2023) similarly, they are likely to enjoy similar future releases like Azaad (2025) or Deva (2025).
- Scaling Up – In practical applications (Netflix, Prime Video), machine learning models process large datasets using deep learning (e.g., neural networks) for more accurate, personalized recommendations.
By combining mathematical techniques with ML models, recommendation engines enhance user experience by suggesting the best movies based on real data. 🚀🍿
Justification of the Statement: “If two users rated movies like Animal (2023) and Dunki (2023) similarly, they are likely to enjoy similar future releases like Azaad (2025) or Deva (2025).”
This statement is justified based on the principles of collaborative filtering and cosine similarity in machine learning and recommendation systems. Here’s why:
1️⃣ Concept of User Similarity in Recommendations
- If User A and User B have rated movies like Animal (2023) and Dunki (2023) similarly, their preferences are likely aligned.
- When a new movie (Azaad (2025) or Deva (2025)) is released, if one of them likes it, the other user is statistically likely to enjoy it too.
This forms the basis of collaborative filtering, where recommendations are made based on patterns in user behavior.
2️⃣ Mathematical Justification: Cosine Similarity
We can calculate cosine similarity between users’ rating vectors:
Movie | Animal (2023) | Dunki (2023) | Azaad (2025) | Deva (2025) |
---|---|---|---|---|
User A | 5 | 4 | ? | ? |
User B | 5 | 4 | 5 | 4 |
If the similarity score (cosine similarity) between User A and User B is close to 1, then their future ratings for Azaad (2025) and Deva (2025) are likely to be very similar.
3️⃣ Real-World Examples: How Netflix and Amazon Prime Use This
- Platforms like Netflix, Prime Video, and Hotstar use collaborative filtering + deep learning models to recommend content.
- If you like action-thrillers (Animal), the system suggests similar highly-rated action movies (e.g., Deva).
- If you like emotional or patriotic movies (Dunki), you may get recommendations for period dramas (Azaad).
4️⃣ Machine Learning Implementation
In real-world ML models, we would use:
✅ Collaborative Filtering (User-User or Item-Item Similarity)
✅ Content-Based Filtering (Movie Genre, Cast, Director, etc.)
✅ Hybrid Models (Combining Both Approaches with Deep Learning)
For example, a Neural Collaborative Filtering (NCF) model would predict ratings for Azaad (2025) or Deva (2025) based on historical data of similar users.
Conclusion
✔️ If two users have similar past preferences, they are more likely to enjoy similar movies in the future.
✔️ This is the core principle behind movie recommendation engines used by Netflix, Prime Video, and YouTube.
✔️ Mathematical metrics (cosine similarity) + Machine Learning (collaborative filtering) provide accurate movie suggestions.